In the last decade, the explosive growth of vision sensors and video content has driven numerous application demands for automating human action detection in space and time. Aside from reliable precision, vast realworld scenarios also mandate continuous and instantaneous processing of actions under limited computational budgets. However, existing studies often rely on heavy operations such as 3D convolution and fine-grained optical flow, therefore are hindered in practical deployment. Aiming strictly at a better mixture of detection accuracy, speed, and complexity for online detection, we customize a cost-effective 2D-CNN-based tubelet detection framework coined Accumulated Micro-Motion Action detector (AMMA). It sparsely extracts and fuses visual-dynamic cues of actions spanning a longer temporal window. To lift reliance on expensive optical flow estimation, AMMA efficiently encodes actions’ short-term dynamics as accumulated micro-motion from RGB frames on-the-fly. On top of AMMA’s motion-aware 2D backbone, we adopt an anchor-free detector to cooperatively model action instances as moving points in the time span. The proposed action detector achieves highly competitive accuracy as state-of-the-arts while substantially reducing model size, computational
cost, and processing time (6 million parameters, 1 GMACs, and 100 FPS respectively), making it much more appealing under stringent speed and computational constraints. Codes are available on https://github.com/alphadadajuju/AMMA.
Accumulated micro-motion representations for lightweight online action detection in real-time
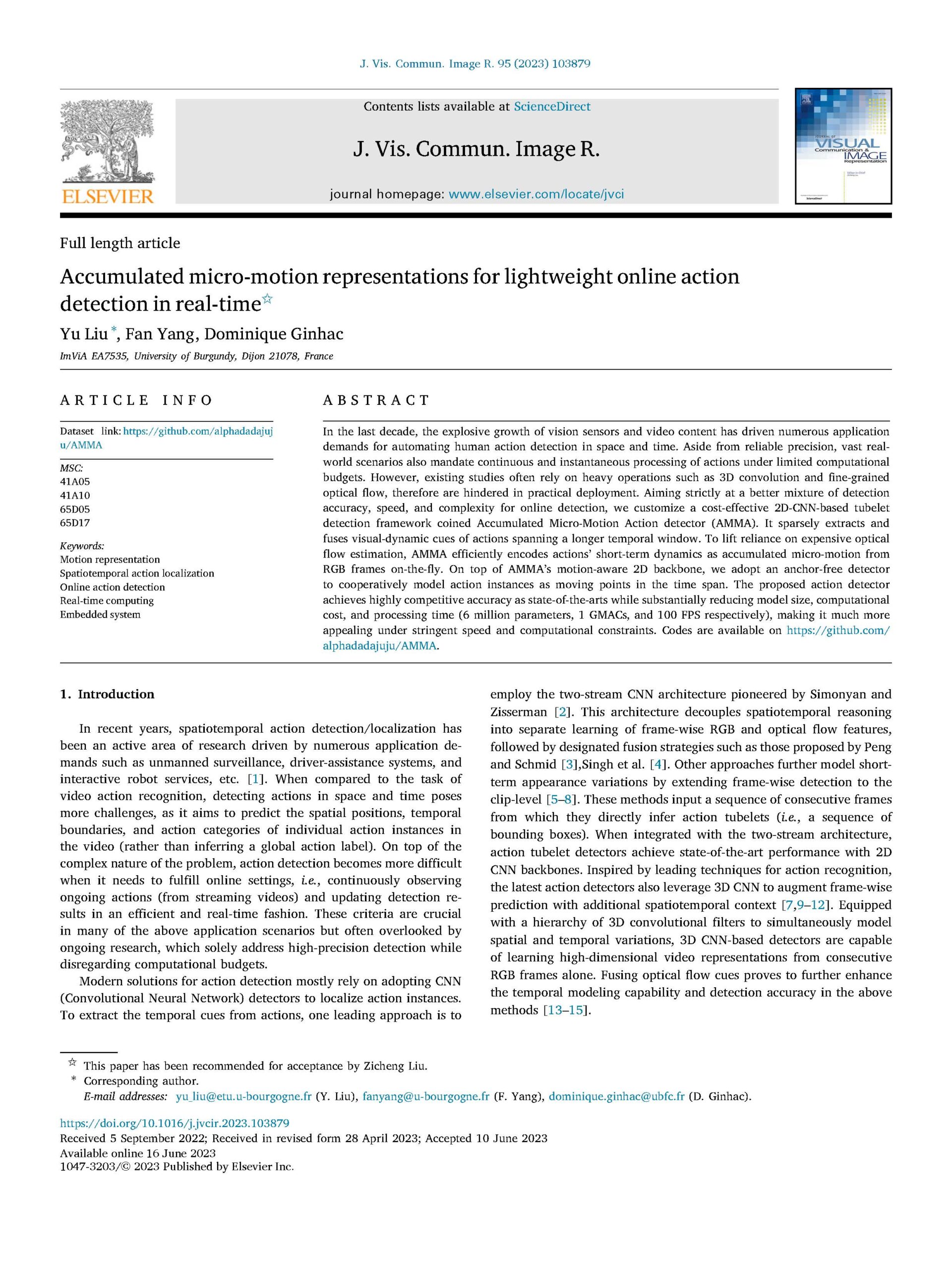